Automotive edge Analytics will help collect, analyze and process data in real time
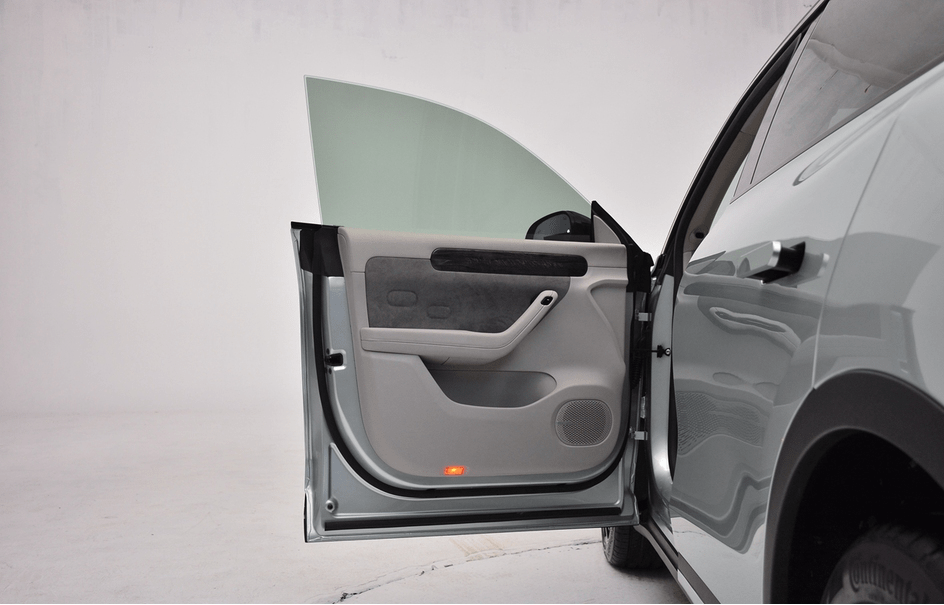
With the use of iot, connected applications and systems are migrating to the cloud, and the number of end devices and their data generated in the cloud is also increasing. In the robotics and iot ecosystem, due to the lack of reliable connectivity, latency and cloud processing, edge devices such as sensors, mobile devices, wearables and many other connected devices will generate a large amount of decentralized data. Companies are using edge analytics and cloud computing to address this challenge. The portfolio brings computing power near the data source to reduce analytics latency and provide real-time opinions and solutions to problems across industries, bringing stability to iot networks. In other words, edge analysis brings data into the algorithm and provides important opinions when data cannot be brought into the algorithm.
How has edge analysis evolved over the years?
Thanks to advances in semiconductor technology,in recent years, MCUS have facilitated faster edge analysis by deploying advanced machine learning methods, such as deep neural networks or convolutional neural networks, with processors with more processing power, special hardware components, and computing power. TensorFlow, keras, and Caffe can be optimized to deploy inference devices such as model Andriod-operated microcontrollers developed on popular frameworks. Inference engines that consider MCU capabilities, such as TensorFlow-Lite, TensorFlow-micro, CMSIS-NN, etc., can perform quantized models at the edge for faster analysis.
Edge analytics helps organizations that need data insights. Let's take a look at how edge analytics can help many industries around the world.
How to strengthen edge analysis?
According to the "Global Automotive Sensor Technology Market", the average number of sensors used in cars has increased from 50-60 to 100+, and in the near future, it will reach 20,000 +, which will generate a large amount of data. For mobile cars, continuous and reliable cloud connectivity is another challenge. For example, if a self-driving car delays sending data to the cloud, data analytics and execution actions could have a significant impact on the success or failure of the self-driving car. Automotive Edge Analytics will help companies collect, analyze, and process data in real time so that necessary actions can be taken immediately. In addition, through artificial intelligence and machine learning, intelligent applications of edge design, such as collision, traffic routes, out-of-sight road detection systems, etc. This ensures optimized asset use, low maintenance, and passenger safety.
How is Edge Analytics revolutionizing health care?
Medical devices powered by the Internet of Things can collect patient data. In the absence of a continuous network connection, edge analysis of the collected data can be performed. As semiconductor technology advances, hardware and machine learning become more efficient, so edge devices can monitor and analyze more complex parameters such as neural activity, heart rate, and blood pressure.
Through edge computing, patient management, remote monitoring, inpatient care and health information management. For example, a doctor's mobile/tablet device is the edge between the patient (data source) and the cloud. Clinicians can input patient data on the edge analytics platform to process and display patient data in real time. This helps treat patients more quickly and with less frequency. In addition, secure computing power between the cloud and devices has been improved to protect patient data.
How is edge Analytics changing manufacturing?
At the manufacturer or factory, any production line on edge equipment involves multiple sensors to constantly measure the temperature, humidity, pressure, and other parameters of goods and equipment. Connecting to the cloud and analyzing data takes a long time. Edge computing can process this data for analysis and implement/require changes in the process. ML It also supports prediction and monitoring. Machine learning algorithms can predict equipment failure and schedule maintenance before it occurs, helping to extend its service life, reduce downtime and save overall maintenance costs.
Why is the cloud still at the heart of analytics?
After understanding the advantages of edge analytics, it is important to understand that it does not replace the cloud, but rather complements the cloud with real-time analytics because of its proximity to the data source. Very few processes continue to execute in the cloud.
Training of machine learning algorithms: The development of machine learning algorithms depends on large amounts of data. Before training the model, a number of entities, relationships, and clusters are extracted from the learning process. This can be done in conjunction with a cloud training model.
Processing power and storage capacity: The unlimited scalability of storage and processing power and easy to deploy analytics make cloud analytics irreplaceable. Because cloud analytics can handle more data that could be very useful, because cloud analytics can handle more types of data. For example, it can add historical data to traffic data, or use edge analytics to analyze all outputs from all devices.
In edge analytics, the cloud can use all edge devices connected to a single cloud application. The cloud has a way of managing data and turning it into meaningful predictions and analytics.
How does the coexistence of edge and cloud analytics complement?
Real-time decision making for iot systems remains challenging due to factors such as latency, bandwidth, power consumption, cost, appearance size, and more. This can be overcome by adding artificial intelligence at the edge.
Reduced data bandwidth/transfer utilization: Moving large amounts of data to the cloud for processing can consume high data bandwidth, cause significant latency, and negatively impact critical time applications. To avoid this delay and eliminate the dependency on data bandwidth, data can be processed at the edge.
Eliminate the need for continuous connectivity to the cloud: In the oil, gas or mining industry, employees work at remote stations far from populated areas, so there is no connectivity. In this case, sensors on robots and other edge devices can capture data, analyze data and monitor operational parameters, regardless of whether the value is within the normal range.
Real-time performance and faster processing speed: Edge computing greatly reduces the amount of data that must be sent over the network, which reduces network congestion and speeds up operations. Edge computing is not a process that runs in the cloud, but a process that runs in a computer, an iot device, or an edge server. Bringing computing to the edge of the network can reduce remote communication between clients and servers and get real-time opinions.
Improve data security (closer to the data source and location awareness) : explain that it is better to analyze the camera itself rather than having a security camera transmit its video content to the cloud to analyze certain situations (unknown persons, objects, etc.). Data privacy and security issues related to biometric data are important for using only local data on the device and not sending it over a cloud connection.
Cloud computing and edge computing all depend on different approaches to implementing applications. While they are not discredited, they are complementary. There can be no one solution that fits all solutions. There are few key factors, such as real-time performance, bandwidth cost, data size, and application complexity. These factors determine edge analytics or cloud analytics, or both.
Die Produkte, an denen Sie interessiert sein könnten
![]() |
PIM300A6Z | DC DC CONVERTER -48V 300W | 7686 More on Order |
![]() |
AXH010A0D | DC DC CONVERTER 2V 20W | 6030 More on Order |
![]() |
EVK011A0B41-HZ | DC DC CONVERTER 12V 132W | 2106 More on Order |
![]() |
EBVW025A0B9641-PZ | DC DC CONVERTER 12V 300W | 2718 More on Order |
![]() |
ATM020A0X43-SR | DC DC CONVERTER 0.75-2V 40W | 4050 More on Order |
![]() |
QRW035A0F41Z | DC DC CONVERTER 3.3V 116W | 7740 More on Order |
![]() |
QRW025A0M41-H | DC DC CONVERTER 1.5V 38W | 7758 More on Order |
![]() |
EQW006A0B1-SZ | DC DC CONVERTER 12V 72W | 6588 More on Order |
![]() |
EQW020A0F1-SZ | DC DC CONVERTER 3.3V 66W | 151 More on Order |
![]() |
ATH030A0X3Z | DC DC CONVERTER 0.8-3.6V 108W | 6714 More on Order |
![]() |
MW005A | DC DC CONVERTER 5V 5W | 5022 More on Order |
![]() |
LW015A91 | DC DC CONVERTER 5V 15W | 4824 More on Order |
![]() |
EQW012A0A1-S | DC DC CONVERTER 5V 60W | 5256 More on Order |
![]() |
AXH003A0X4-SR | DC DC CONVERTER 0.8-3.6V 10W | 4392 More on Order |
![]() |
ATH030A0X3 | DC DC CONVERTER 0.8-3.6V 108W | 4644 More on Order |
![]() |
QBDW025A0B41Z | DC DC CONVERTER 12V 300W | 6732 More on Order |
![]() |
QBVW033A0B841-PZ | DC DC CONVERTER 12V 400W | 7812 More on Order |
![]() |
EBDW020A0B841-HZ | DC DC CONVERTER 12V 240W | 6102 More on Order |
![]() |
QBVW033A0B541Z | DC DC CONVERTER 12V 400W | 5814 More on Order |
![]() |
ESTW015A0A641Z | DC DC CONVERTER 5V | 2160 More on Order |
![]() |
KHHD010A0F41-SRZ | DC DC CONVERTER 3.3V 33W | 5238 More on Order |
![]() |
PDT012A0X3-SRDZ | DC DC CONVERTER 0.45-5.5V | 7200 More on Order |
![]() |
NSR020A0X43Z | DC DC CONVERTER 0.6-6V 120W | 7182 More on Order |
![]() |
SHHD003A0A41-SRZ | DC DC CONVERTER 5V 15W | 17856 More on Order |