How does a self-driving system sense its surroundings?
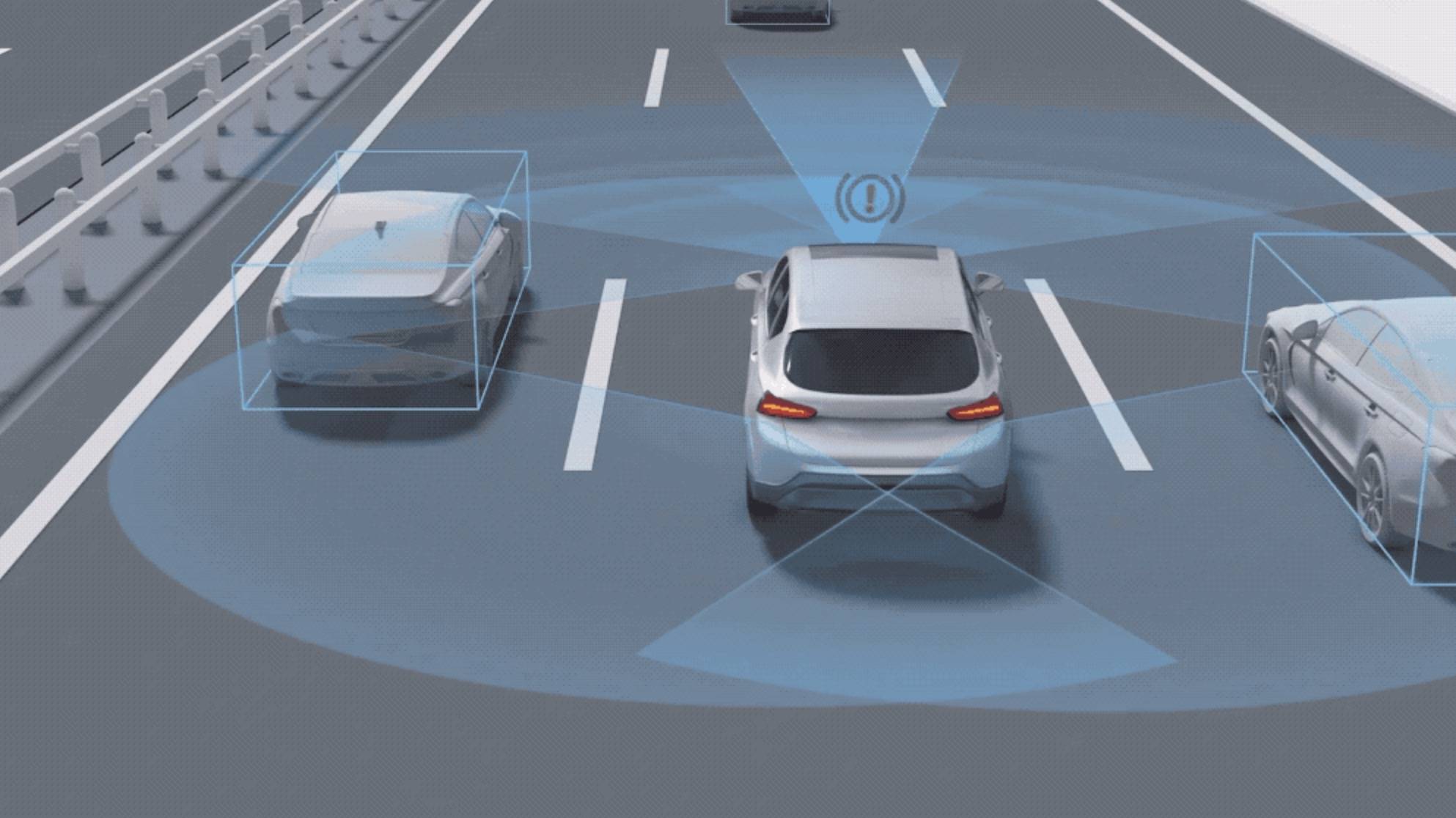
Ordinary people may have questions about the current popularity of autonomous driving. How does the autonomous driving system perceive the surrounding environment and make appropriate decisions and implementation? In fact, there are three main modules of autonomous driving technology, namely perception, planning and control.
They cooperate with each other to ensure the safety and comfort of the vehicle. Simply put, perception is composed of environment modeling and localization, which rely on external and ontological sensors respectively. The plan aims to generate the best trajectory based on the information conveyed by the perceived results in order to reach a given destination. Finally, the control module is dedicated to tracking the generated trajectory by commanding the vehicle's actuators.
Among them, perception module, as the "eyes" of autonomous driving, is the main technology of vehicle detection, and is also the basis and premise of autonomous driving.
As the most upstream of the software stack, the perception module is the most popular direction in the field of autonomous driving. Only by maturely solving this problem can autonomous driving climb the mountain and continue to attack the downstream decision-making brain domain. This article will guide the reader through the most critical perception detection techniques in detail.
I, what is the perception module of autonomous driving?
Sensing is the process of collecting and processing data collected by various hardware sensors and generating real-time sensing results. The sensor types of the sensing layer generally include lidar, camera, millimeter wave radar, ultrasonic radar, speed and acceleration sensors. For different sensors, they have different advantages and characteristics, and most autonomous driving needs to be equipped with a variety of sensors in order to perceive external objects as perfectly as possible in a complex world.
Lidar (LiDDAR) : It can release multiple laser beams, receive the reflected signal of the object, and calculate the distance between the target and itself. Lidar provides ambient D point cloud images of the three generated environments and provides a series of point cloud images (x, y, z) with coordinates that can locate vehicles very accurately compared to those on existing high-precision maps. Lidar independently emits light and collects reflected signals, so it can work in nighttime environments. But bad weather conditions, such as snow and fog, can affect the laser and reduce accuracy.
Millimeter wave radar: Millimeter wave radar emits and receives electromagnetic waves. Compared with LiDAR, millimeter wave radar has many scenarios for measuring short distances, such as side warning, reverse warning, etc. Round-the-clock work is essential, but the resolution is low and imaging is difficult. Millimeter wave technology is very mature compared to liDAR and has been used for adaptive cruising since the 1990s. Millimeter wave radar has the absolute advantage of penetrating dust fog, snow and wind, and is not affected by bad weather, and has become an indispensable main sensor for autonomous driving.
Camera: the "eyes" of automatic driving, recognizing signs and objects, but not dot matrix modeling, long-distance ranging. Camera technology is the most mature, the earliest automotive applications, is the absolute mainstream vision sensor in the ADAS stage. Entering the era of autonomous driving, thanks to the camera's unique visual image recognition function, it is a true autonomous driving eye. Depending on the integration of the multi-sensor system, at least six cameras are required. At present, it is not easy for new entrants to gain a competitive advantage due to the advantages of cost, technology and customers.
Having an object that each sensor senses, the next step is to fuse it.
II.Perceptual fusion technology
Automatic analysis and synthesis to complete the information processing required for decision making and estimation. Similar to human perception, different sensors have functions that other sensors cannot replace. When various sensors perform multi-level and multi-space information complementation and optimization combination processing, the observation environment is finally interpreted in a consistent manner.
The difficulty of multi-sensor integration technology and project implementation is undoubtedly complex, so why are many autonomous driving companies still flocking to overcome the problems of project implementation? This is because multi-sensor integration can make good use of the advantages of each sensor itself to output more stable and comprehensive perceptual information for the downstream after unification, so that the downstream control module can achieve the final safe driving of the vehicle according to these accurate and stable results. It can be said that a good perceptual integration module will greatly improve the accuracy and safety of decision-making, and will directly represent the final level of intelligent performance of autonomous driving.
III.Technical classification of perception module
Unmanned vision perception system is based on neural network deep learning vision technology and applied to the field of unmanned driving. It is mainly divided into four modules: DynamicObjectDetection (DOD), passing space (FS), lane detection (LD). LaneDetection (SOD), StaticObjectDetection (SOD).
1. DynamicObjectDetection (DOD: DynamicObjectDetection)
The purpose of dynamic object detection is to identify vehicles (cars, trucks, electric vehicles, bicycles), pedestrians and other dynamic objects. Detection difficulties include detection category, multi-target tracking, distance measurement accuracy, complex external environmental factors, shielding, and different directions; Pedestrian, vehicle type, difficult to cover, easy to misdetect; Added challenges such as tracking and pedestrian identity switching.
2. Traffic space (FS: FreeSpace)
Spatial detection is to divide the safety boundary (driving area) of vehicles, mainly for vehicles, ordinary road edges, side stone edges, visible boundaries without obstacles, unknown boundaries, etc. The difficulties of testing include complex environmental scenarios, complex boundary shapes and diversity, resulting in difficulty in generalization.
Unlike other types of detection that have a clear single type of detection (such as vehicles, pedestrians, traffic lights), traffic space requires accurate demarcation of the driving safety zone and the boundaries of obstacles that affect the vehicle's progress. However, when the vehicle acceleration and deceleration, road bumps, up and down the ramp, the camera pitch Angle will change, the original camera calibration parameters are no longer accurate, the ranging error after the projection of the world coordinate system is large, and the traffic space boundary shrinks or opens.
3. LaneDetection (LD: LaneDetection)
The purpose of lane detection is to detect a variety of lane lines (single/double lane lines, solid lines, dashed lines, double lines), as well as linear colors (white/yellow/blue) and special lane lines (conjunctions, deceleration lines, etc.). At present, it can be maturely applied in the field of assisted driving.
At the same time, there are many difficulties in lane detection, including many types of routes and irregular road surface detection. In case of ground water, invalid signs, road repair, shadow, lane lines, easy to false detection, missed detection. It is difficult to fit curved lane lines, far end lane lines and roundabout lane lines, and the detection results are easy to blur.
4. Static object detection (SOD: StaticObjectDetection)
Static object detection is the detection and recognition of static objects such as traffic lights and traffic signs. It's also one of the most common objects in autonomous driving. Among them, traffic lights and traffic signs belong to small object detection, and the pixel ratio in the image is very small, especially at long-distance intersections, which is more difficult to identify.
Visual detection, under bright light, sometimes it is difficult for the human eye to recognize, and the car stopped in front of the zebra crossing needs to correctly identify the traffic light in order to make the next judgment. There are many kinds of traffic signs, and the amount of collected data is easy to be uneven, resulting in imperfect training of detection models.
Iv. Conclusion
From the above summary of the current perception module, the current environment perception technology has greatly improved than before, as can be seen from the current landing degree of assisted driving. But at the same time, in order to achieve true unmanned driving, the accuracy and stability of visual detection need to be achieved, which is a huge challenge for the perception module. Due to the natural flaws of deep learning algorithms, how to deal with objects that have never been seen before, and how to deal with the Cornercase of objects in extreme scenes, will be a problem that hinders the development of perception. Only by fully addressing these issues can we achieve true driverless driving.
Die Produkte, an denen Sie interessiert sein könnten
![]() |
62045-P2S02 | XFRMR TOROIDAL 10VA CHAS MOUNT | 2430 More on Order |
![]() |
62044-P2S02 | XFRMR TOROIDAL 10VA CHAS MOUNT | 6048 More on Order |
![]() |
62043-P2S02 | XFRMR TOROIDAL 10VA CHAS MOUNT | 5472 More on Order |
![]() |
62035-P2S02 | XFRMR TOROIDAL 7VA CHAS MOUNT | 6642 More on Order |
![]() |
62034-P2S02 | XFRMR TOROIDAL 7VA CHAS MOUNT | 7938 More on Order |
![]() |
62025-P2S02 | XFRMR TOROIDAL 5VA CHAS MOUNT | 3924 More on Order |
![]() |
62021-P2S02 | XFRMR TOROIDAL 5VA CHAS MOUNT | 5058 More on Order |
![]() |
62012-P2S02 | XFRMR TOROIDAL 3.2VA CHAS MOUNT | 3204 More on Order |
![]() |
62075-P2S02 | XFRMR TOROIDAL 35VA CHAS MOUNT | 7308 More on Order |
![]() |
62073-P2S02 | XFRMR TOROIDAL 35VA CHAS MOUNT | 5292 More on Order |
![]() |
70074K | XFRMR TOROIDAL 35VA THRU HOLE | 6840 More on Order |
![]() |
70054K | XFRMR TOROIDAL 15VA THRU HOLE | 4716 More on Order |
![]() |
70015K | XFRMR TOROIDAL 3.2VA THRU HOLE | 7578 More on Order |
![]() |
70014K | XFRMR TOROIDAL 3.2VA THRU HOLE | 5562 More on Order |
![]() |
70013K | XFRMR TOROIDAL 3.2VA THRU HOLE | 4392 More on Order |
![]() |
70003K | XFRMR TOROIDAL 1.6VA THRU HOLE | 2520 More on Order |
![]() |
70002K | XFRMR TOROIDAL 1.6VA THRU HOLE | 5040 More on Order |
![]() |
62082-P2S02 | XFRMR TOROIDAL 50VA CHAS MOUNT | 4986 More on Order |
![]() |
62033-P2S02 | XFRMR TOROIDAL 7VA CHAS MOUNT | 6480 More on Order |
![]() |
70005K | XFRMR TOROIDAL 1.6VA THRU HOLE | 7218 More on Order |
![]() |
AC1200 | CURR SENSE XFMR 200A T/H | 2142 More on Order |
![]() |
AC1050 | CURR SENSE XFMR 50A T/H | 7362 More on Order |
![]() |
AC1015 | CURR SENSE XFMR 15A T/H | 5166 More on Order |
![]() |
AC1010 | CURR SENSE XFMR 10A T/H | 5963 More on Order |